Can machine learning—the branch of artificial intelligence that has made possible self-driving cars and that credit card companies use to identify potential fraud—help transform cancer care? Computer scientist David Sontag, PhD, is trying to find out.
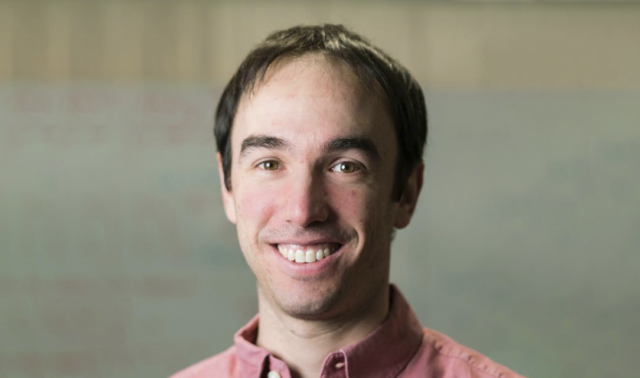
Although cancer is never easy, some types are, relatively speaking, easy to treat: they behave in predictable ways and generally respond well to established treatments. But others pose a series of clinical conundrums, as physicians and patients must cope with a wide range of genetic variants, presentations, prognoses, complications and treatment options. Because standard clinical guidelines cannot fully address this diversity, physicians and patients must make treatment decisions that are, to some degree, educated guesses.
A potential solution to this difficulty, says Sontag, is to harness the power of machine learning.
In this branch of artificial intelligence, computers aren’t given rigid instructions for solving problems, but rather are “taught” to develop their own algorithms to classify data and make predictions. With the support of The Mark Foundation, Sontag is exploring whether machine learning can help guide the treatment of multiple myeloma, for which the five-year survival rate barely exceeds 50%.
“Machine learning has been used for decades to create models for risk stratification of cancer progression,” notes Sontag, who is professor of electrical engineering and computer science at the Massachusetts Institute of Technology. “But we’re trying to take these models to the next level, by incorporating rich clinical and biological data and by additionally predicting treatment response.”
Sontag’s artificial intelligence work—which fuses two of his long-time passions, computer science and improving people’s well-being—has previously led to the development of machine learning algorithms that predict the presence undiagnosed diabetes or the course of chronic obstructive pulmonary disease. He decided to focus on multiple myeloma for his initial foray into cancer research in part because of the nature of the disease. “Patients differ in their genetic abnormalities, the expression of those genes, and the drugs they respond to,” Sontag notes.
Tackling a disease with such heterogeneity presented him with an intriguing challenge that was only heightened by the recent development of new treatments whose potential for specific patients is not yet well understood. In a sense, a more straightforward, easier-to-treat type of cancer would not have been a sufficiently rigorous test of machine learning’s potential.
But multiple myeloma was also on Sontag’s radar because his mother, Frances David-Sontag, was diagnosed with it in 2014. To educate himself about her situation, he attended a conference for myeloma patients and spoke with experts. Ultimately, his goal was to “develop an algorithm that could predict response to treatment given what we know about a patient,” he explained. “I wanted to have it ready so that I could help guide the choice of therapy for my mother.” Sadly, Sontag’s mother’s died in 2017, just as his efforts were getting underway. Moreover, he still faced two major obstacles.
The first was finding a suitable database. Machine learning algorithms are fueled by data, and they need a lot of it—ideally, a wide range of genetic, clinical, and demographic data from a huge number of patients. Obtaining sufficient high-quality data is hard for a relatively rare cancer like multiple myeloma (only 30,000 cases are diagnosed each year), but a 1,000-patient dataset from the prospective CoMMpass study is providing some initial fodder for Sontag’s algorithms.
The other challenge was getting funding. “The Mark Foundation was transformational for getting this work off the ground,” Sontag says. “The research I’m doing here is not the type that is traditionally funded by the other foundations and corporate sponsors that support my work.” For a while, Sontag was able to pursue the myeloma research only as a side project. “But once we got the funding, I was able to hire two PhD students to work with me to go much, much deeper than we had been able to do before.”
Sontag acknowledges that the project is risky. “That’s partly because modeling disease progression is extremely difficult—even if you ignore the additional complexities that I’m adding. Moreover, it’s not yet clear that machine learning methods will work with a dataset of 1,000 patients, even though it’s one of the largest datasets of its type. In addition, our research seeks to change the paradigm of progression modeling to be more holistic, so that it predicts a whole range of possible outcomes for patients, such as future symptoms caused by cancer progression or side effects of treatment. That hasn’t been done before.”
But if the five-year project is successful, the benefits could be considerable. If successful, the models that Sontag and his colleagues are creating may “go substantially beyond the current state of the art in forecasting disease progression and treatment response.” Because “only a handful of researchers are currently working on disease progression models,” Sontag hopes to create a community of likeminded investigators who can support each other’s efforts and establish benchmarks for success. Another goal of the research is to lay the groundwork for the development of systems that use artificial intelligence to coordinate the full range of patients’ health care—an approach that perhaps could have benefited Sontag’s mother, who ultimately died from a myeloma complication (amyloidosis) that might have been diagnosed and addressed earlier if the various specialists who were treating her had been fully cognizant of the range of symptoms she was experiencing.
Finally, the project’s success will require what Sontag calls “translational work”—the conversion of the algorithm’s predictions to a form that doctors and patients can understand and can use to make decisions and improve outcomes. “If we succeed,” he says, “it will really accelerate progress in treating this disease.”